AutoGen是微软研究团队开发的人工智能(AI)智能体创建和管理工具。它为开发者提供了一个框架,帮助他们创建和管理 AI 智能体。AutoGen 旨在为开发者提供丰富的功能和灵活的控制,使他们能够根据特定需求定制智能体的行为和功能。
这个工具为开发者提供了一个强大的平台,帮助他们在人工智能领域创建和管理智能体。通过 AutoGen,可以彼此对话的多个代理来共同处理各种任务,从而促进大型语言模型 (LLM) 应用程序的创建。
autogen 相对其他 agent 框架更大优势在于用户可介入具体过程,多个 agent 可交互,groupagent 等。
Autogen 基本结构
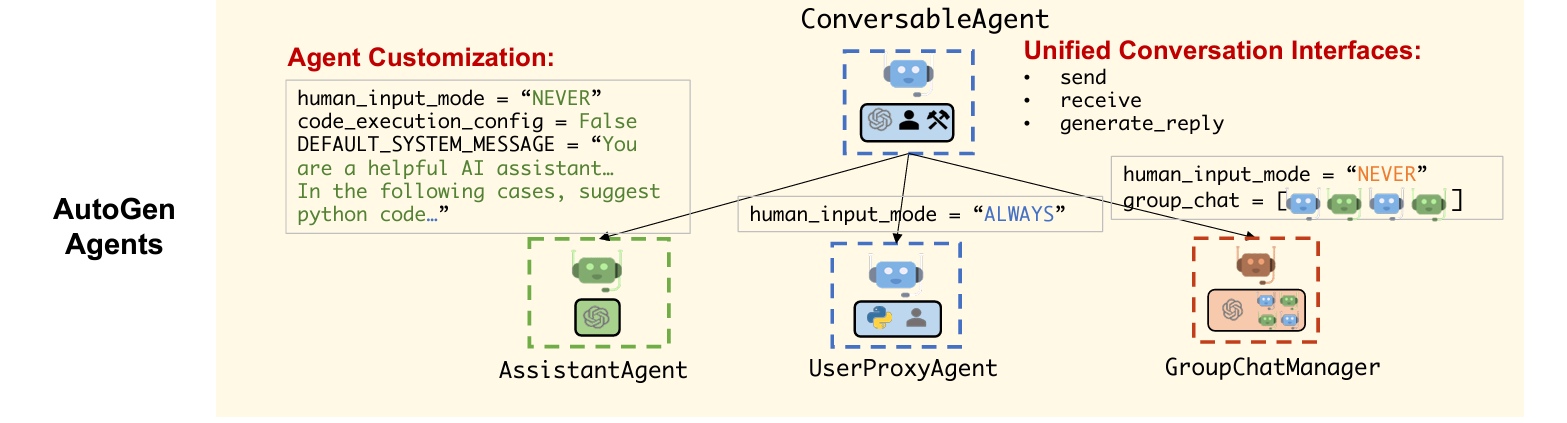
Autogen 首先定义了一个泛型 ConversableAgent 类,这些代理能够通过交换消息来相互交谈以共同完成任务。代理可以与其他代理通信并执行操作。不同的代理在接收消息后执行的操作可能不同。
两个具有代表性的子类是 AssistantAgent 和 UserProxyAgent :
- AssistantAgent AssistantAgent 是 ConversableAgent 的一个子类,具有以下特点:
- 配置了默认的系统消息,用于使用语言模型解决任务。
- 默认的系统消息包括建议 Python 代码块和调试信息。
- 默认情况下,
human_input_mode
设置为“NEVER”,而code_execution_config
默认为 False。 - 代理不执行代码,而是期望用户执行代码。
- UserProxyAgent UserProxyAgent 是 ConversableAgent 的子类,具有以下特点:
- 可以执行代码,并向其他代理提供反馈。
- 参数
human_input_mode
默认设置为“ALWAYS”,而llm_config
默认设置为 False。 - 默认情况下,代理在每次收到消息时会提示人工输入。在本例中,我们将
human_input_mode
默认设置为“NEVER”,即无需人工参与。
这两个子类提供了不同的功能和行为,适用于不同的应用场景和用户需求。通过合理配置这些子类,可以实现更高效和智能的对话交互体验。
3. autogen 实现包含搜索和爬取的 researcher
#pip install pytube pyautogen openai langchain serpapi google-search-results pyppeteer langchain_openai
import os
import json
import requests
import autogen
import openai
from bs4 import BeautifulSoup
os.environ["OPENAI_API_KEY"] = openai_api_key
config_list = [
{
"model": "gpt-3.5-turbo-1106",
"api_key": openai_api_key
}
]
#要用后面版本的 api, 可以设置 api preview。因为前面没有 function_call,会报错
定义两个 function:
- 利用 serpapi 的 google 搜索
- 构建自建的 browserless 服务,如何自建的 browserless 服务,进行爬虫任务。
def search(query: str):
from serpapi import GoogleSearch
params = {
"engine": "google",
"q": query,
"api_key": serper_api_key
}
search = GoogleSearch(params)
results = search.get_dict()
organic_results = results["organic_results"]
return json.dumps(organic_results)
def scrape(url: str):
# scrape website, and also will summarize the content based on objective if the content is too large
# objective is the original objective & task that user give to the agent, url is the url of the website to be scraped
print("Scraping website...")
# Define the headers for the request
headers = {
'Cache-Control': 'no-cache',
'Content-Type': 'application/json',
}
# Define the data to be sent in the request
data = {"url": url}
# Convert Python object to JSON string
data_json = json.dumps(data)
# Send the POST request
#If using the browserless API, please utilize the URL https://chrome.browserless.io.
#post_url = "https://chrome.browserless.io/content?token=2db344e9-a08a-4179-8f48-195a2f7ea6ee"
post_url = "http://ip:port/chrome/content"
response = requests.post(post_url, headers=headers, data=data_json)
# Check the response status code
if response.status_code == 200:
soup = BeautifulSoup(response.content, "html.parser")
text = soup.get_text()
print("CONTENT:", text)
if len(text) > 8000:
output = summary(text)
return output
else:
return text
else:
print(f"HTTP request failed with status code {response.status_code}")
#content = scrape("https://aigonna.com/2021/08/23/NLP%20Paper%2025.NEZHA%20%E8%AE%BA%E6%96%87%E7%AC%94%E8%AE%B0/")
from autogen import config_list_from_json
from langchain.agents import initialize_agent
from langchain.chat_models import ChatOpenAI
from langchain.text_splitter import RecursiveCharacterTextSplitter
from langchain.chains.summarize import load_summarize_chain
from langchain import PromptTemplate
def summary(content: str):
llm = ChatOpenAI(temperature=0, model="gpt-3.5-turbo-16k-0613")
text_splitter = RecursiveCharacterTextSplitter(separators=['\n\n', '\n'],
chunk_size=10000,
chunk_overlap=500
)
docs = text_splitter.create_documents([content])
map_prompt = """Write a detailed summary of the following text for a research purpose:"{text}"SUMMARY:"""
map_prompt_template = PromptTemplate(
template=map_prompt,
input_variables=['text']
)
summary_chain = load_summarize_chain(
llm=llm,
chain_type='map_reduce',
map_prompt=map_prompt_template,
verbose=True
)
output = summary_chain.run(input_documents=docs)
return output
researcher 主要功能:
- 首先对 Researcher(在这里被命名为 researcher)进行了配置,包括其名称、系统消息和执行的功能。系统消息定义了 Assistant Agent 的目标,而功能列表定义了 Assistant Agent 可以执行的操作,包括 ” 搜索 ” 和 ” 抓取 ”。
- 然后创建了一个名为 UserProxy 的代理,这个代理负责管理用户的输入和代码执行配置。它还定义了一个函数映射,将 ”search” 和 ”scrape” 映射到对应的函数上。
- 通过 UserProxy,使用用户的查询信息初始化与 Research Agent 的对话。
- 然后通过 UserProxy,设置接收者为 Researcher,获取研究报告的摘要。
- 最后,函数返回了最后一个由代理收到的消息,返回研究报告的摘要和参考链接。
def research(query):
llm_config_researcher = {
"functions": [
{
"name": "search",
"description": "google search for relevant information",
"parameters": {
"type": "object",
"properties": {
"query": {
"type": "string",
"description": "Google search query",
}
},
"required": ["query"],
},
},
{
"name": "scrape",
"description": "Scraping website content based on url",
"parameters": {
"type": "object",
"properties": {
"url": {
"type": "string",
"description": "Website url to scrape",
}
},
"required": ["url"],
},
},
],
"config_list": config_list}
researcher = autogen.AssistantAgent(
name="researcher",
system_message="Research about a given query, collect as many information as possible, and generate detailed research results with loads of technique details with all reference links attached; Add TERMINATE to the end of the research report;",
llm_config=llm_config_researcher,
)
user_proxy = autogen.UserProxyAgent(
name="User_proxy",
code_execution_config={"last_n_messages": 2, "work_dir": "research"},
is_termination_msg=lambda x: x.get("content", "") and x.get("content","").rstrip().endswith("TERMINATE"),
human_input_mode="TERMINATE",
function_map={
"search": search,
"scrape": scrape,
}
)
user_proxy.initiate_chat(researcher, message=query)
# set the receiver to be researcher, and get a summary of the research report
user_proxy.stop_reply_at_receive(researcher)
user_proxy.send("Give me the research report that just generated again, return ONLY the report & reference links", researcher)
# return the last message the expert received
return user_proxy.last_message()["content"]
query = "autogen usage"
research(query)
参考
正文完